K. Brent Venable
Professor
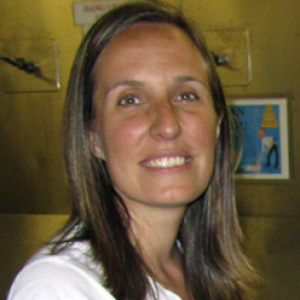
Biography
K. Brent Venable currently holds a joint appointment as professor of computer science at Tulane University and research scientist at IHMC. Her main research interests are within artificial intelligence and regard, in particular, constraints, preferences, temporal reasoning and computational social choice. In particular, her research is dedicated to providing a solid framework for the design and deployment of intelligent systems able to reason about preferences. Before joining Tulane and IHMC, she was a tenured assistant professor of computer science at the University of Padova, Italy. She received her doctorate in computer science and also the Laurea Magna cum Laude in Mathematics from University of Padova. Dr. Venable is the co-author of a book, “A Short Introduction to Preferences: Between Artificial Intelligence and Social Choice”, Morgan & Claypool (2011), and has published over seventeen journal papers and sixty conference papers. Dr. Venable maintains a lively collaboration with several research centers, among which NICTA and NASA Ames.
Education
University of Padova
University of Padova
Articles
Heuristic Strategies in Uncertain Approval Voting Environments
2019
In many collective decision making situations, agents vote to choose an alternative that best represents the preferences of the group. Agents may manipulate the vote to achieve a better outcome by voting in a way that does not reflect their true preferences. In real world voting scenarios, people often do not have complete information about other voter preferences and it can be computationally complex to identify a strategy that will maximize their expected utility.
Learning Preferences in a Cognitive Decision Model
2019
Understanding human decision processes has been a topic of intense study in different disciplines including psychology, economics, and artificial intelligence. Indeed, modeling human decision making plays a fundamental role in the design of intelligent systems capable of rich interactions.
Multi-agent soft constraint aggregation via sequential voting: theoretical and experimental results
2019
We consider scenarios where several agents must aggregate their preferences over a large set of candidates with a combinatorial structure. That is, each candidate is an element of the Cartesian product of the domains of some variables (i.e., features). These scenarios are very common when candidates are described by feature vectors, such as cars, or houses, or any complex product.